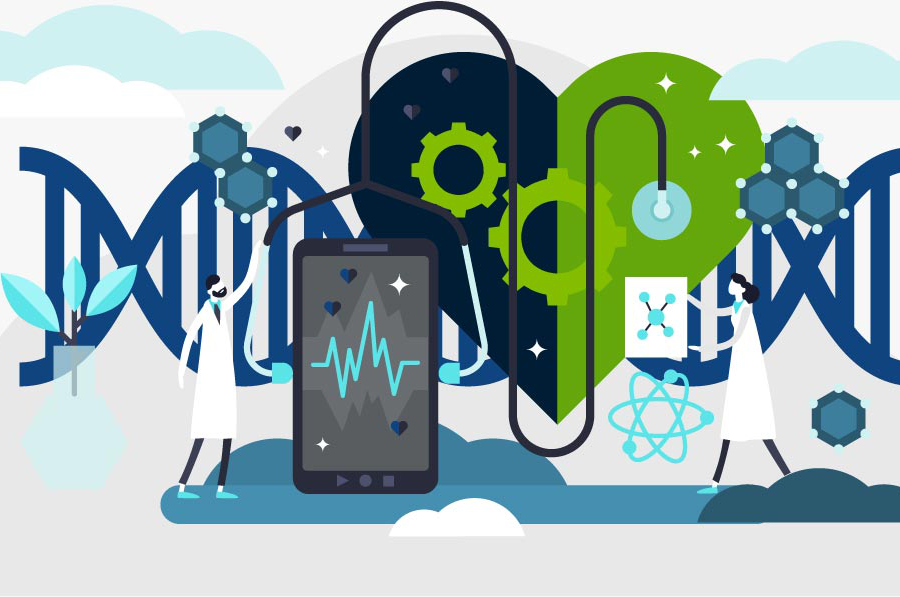
Harnessing the potential of patient data using artificial intelligence
Healthcare resources are severely stretched. And although a lot of patient data has been collected, making use of it is difficult. New tools are needed to ease the workload of healthcare personnel. This is where artificial intelligence comes in: AI can process large amounts of patient data in an instant to aid decision-making.
Effective use of patient data is important for the quality of care and resource management. The power of patient data can be harnessed by extracting information using various data processing methods, or by using AI models that learn from the data.
For example, it is possible to predict a patient’s risk of developing heart failure or stroke using an application that analyses patient data. This can then be used for screening or to formulate a proposal for action. There are various means of calculating risks, such as conventional risk calculators based on cohort studies, and artificial intelligence methods that learn from the patient data used.
Making use of patient records
A large proportion of patient data is stored in patient records, in the form of unstructured, i.e., free-form text. These records describe the patient’s background, their symptoms and their development over time, and the treatment the patient has received. However, this information-rich free text is difficult to use, as it is not in a form that computers can understand, and health and social services personnel often do not have the time to read it thoroughly.
In recent years, there have been major developments in artificial intelligence in the field of natural language processing (NLP). ChatGPT, for one, has brought to the public’s attention the enormous progress being made in the field.
The potential uses of NLP models include finding out how much a patient smokes if the information is not recorded in a structured way. There are so many different ways of expressing even such a simple thing that it can be difficult to work it out with a conventional algorithm.
The advantage of AI: flexibility
Conventional risk calculators may not work for a different population than the one for which the original risk assessment study was conducted. In one case, patients in intensive care had a much higher probability of developing the diseases studied than the probability predicted by the risk calculator. Conventional risk calculators are also demanding when it comes to input values: if even one is missing, the risk cannot be calculated. This limits their usefulness for automated processing of patient data, as healthcare data often has gaps and is incomplete.
The advantage of AI-based models is their flexibility. The model can be taught or fine-tuned separately for each application or data set. With AI, there is more choice in terms of input values – you can use what is available. The AI models used in the experiment only used basic patient data and diagnosis codes, but still managed to produce better results than traditional risk calculators.
On the other hand, the challenge of using AI lies in its interpretability and validation and getting healthcare personnel and patients to accept its use. With a complex model, the reasons for any outcome become blurred, and when developing a model, it is important to try to ensure the validity and fairness of the model and data.
AI as part of healthcare
In my view, AI is likely to become a significant part of healthcare processes in the near future. In Finland, the Patient Data Repository of the Kanta Services contains a wealth of usable data on many of us, but much remains to be done in order to make effective use of it. For example, a conversational AI model trained on patient data could make the review of patient histories far more efficient than at present. Research and emerging needs in the field can also help prioritize the development of the repository in terms of the data it stores.
Atostek has just started a research project, enabled by the Act on the Secondary Use of Health and Social Data (552/2019) and the Finnish Social and Health Data Permit Authority Findata, to study the pseudonymized patient data in the Patient Data Repository of the Kanta Services and its suitability for procedures such as automated processing and risk calculation.
In my master’s thesis, I investigated the suitability of patient data for risk calculation using an American critical care database called MIMIC-III, which contains comprehensive data on about 50,000 patients. The data available on the patients includes basic patient data, measurement results, laboratory results, codes for diagnoses and procedures performed, and textual patient records.
Janne Tommola
Software Developer
I started working as a software developer at Atostek in 2019. At the end of 2022, I graduated from the Tampere University with a master’s degree in Information Technology. I have been involved in various projects, with the focus ranging from machine vision and health data research to a design program for ventilation machines, for example. I am particularly interested in designing new things and the possibilities presented by artificial intelligence.